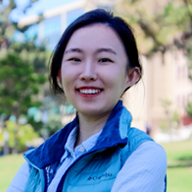
Elizabeth M.Y. Lee
Samueli School of Engineering
University of California, Irvine
Irvine, CA 92697
Elizabeth (Liza) Lee is an assistant Professor of Materials Science and Engineering; and Chemical and Biomolecular Engineering (by courtesy). Her research program is focused on the design of materials with heterogeneous interfaces with applications in energy, sustainability, and quantum applications, and on the use and development of theoretical and computational methods for simulations of materials. Her awards include the DOE Leadership Computing Challenge Award, NSF Graduate Research Fellowship, American Institute of Chemical Engineers Electronics and Photonics Materials Award, the University of Chicago Mentoring Award, the UCI Samueli Faculty Development Chair, and recently, UCI Engineering Student Council’s Professor of the Year.
Ph.D., Massachusetts Institute of Technology, Chemical Engineering
M.S., Massachusetts Institute of Technology, Chemical Engineering Practice
B.S., Johns Hopkins University, Chemical and Biomolecular Engineering
B.A., Johns Hopkins University, Chemistry
Our research focuses on predicting the atomic-scale dynamics and properties of interfaces, which play a critical role in materials and devices for energy conversion, chemical transformation, and nanoscale sensing. These interfaces are often heterogeneous, formed by different phases and classes of materials (e.g., electronic and “hard” vs. dynamic and “soft”). We aim to understand the chemical, electronic, and physical processes that occur at these interfaces using theoretical and computational tools based on ab initio calculations, phenomenological models, and machine learning methods. Our goal is to use insights from our atomistic simulations to rationally design new materials with applications in energy research, circular economy, and quantum information science. Current research activities focus on three areas: (1) quantum defects in semiconductors, (2) surface phenomena in solid-state materials, and (3) computational methods for materials simulations using machine learning approaches.