UCI Engineers Develop Neural Network to Optimize Alloy Compositions for Advanced Energy Systems
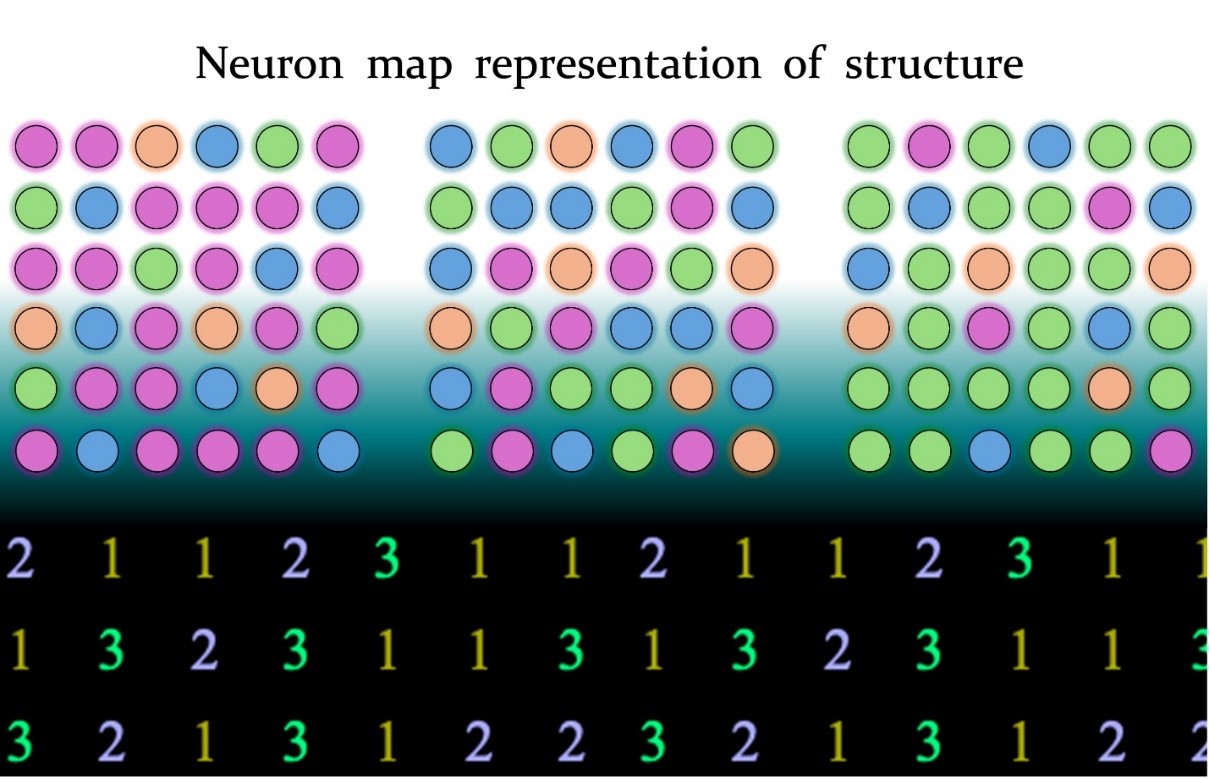
May 15, 2024 - UC Irvine engineering researchers have developed a neural network to search and identify materials with optimal compositions to improve the performance of energy systems.
“Ultrastrong alloys facilitate deployment of fusion reactors and enhance the energy efficiency of turbine engines - both are imperative for the nation,” explained Penghui Cao, assistant professor of mechanical and aerospace engineering who leads the research that appears this month in Nature Communications. “Identifying high-performing materials that better address these critical needs has been a continuous and enduring pursuit.”
Over the past two decades, new metal alloy designs that combine multiple principal elements in high concentration have emerged and triggered a surge in material research. These multicomponent alloys (MCAs), often called high-entropy alloys or multiprincipal element alloys, demonstrate exceptional mechanical properties, including high-temperature strength, putting them in good position for applications in nuclear reactors and propulsion systems. The MCAs, however, have opened up a new frontier in which the composition possibilities are practically limitless.
“The evolution of these multicomponent alloys has profoundly broadened the spectrum of possible alloy systems, making the efficient and accurate exploration of the entire composition space and identifying the desired combinations a formidable challenge,” said Cao. He and UCI doctoral student Bin Xing created a machine learning framework representative of structure and chemistry called the neuron map. The lowest dimensionality of the neuron map as a descriptor allows the neural network model to accurately predict fundamental properties across the entire space. To demonstrate this model, the study predicts the diffusion barrier in the ternary space of chemical elements niobium, molybdenum and tantalum (NbMoTa) alloys and uncovers diffusion-induced local chemical ordering.
“We reveal the diffusion-induced chemical and structural evolution in complex, concentrated chemical environments and find the critical temperature at which the chemical order reaches a maximum,” said Xing.
The UCI team’s neuron map presents an effective process for exploring the vast compositional space of multicomponent alloys and lays the groundwork for discovering ultrastrong and creep-resistant alloys for application in severe environments.
Additional authors include Timothy J. Rupert and Xiaoqing Pan. The research was supported by National Science Foundation Materials Research Science and Engineering Centers (MRSEC) and DOE Basic Energy Sciences.
– Lori Brandt