UCI Engineers Develop a New AI-based Method for Analyzing Echocardiograms
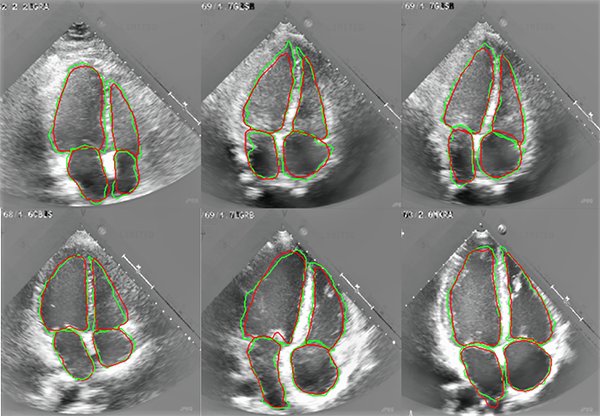
Aug. 27, 2020 - UC Irvine engineers have developed a novel artificial intelligence method for assessing echocardiograms that could help cardiologists more accurately determine heart function and disease.
The machine learning platform will automatically segment the heart’s four chambers and calculate their volumes, eliminating the variability in human assessment of cardiac function, using echocardiography data. The research, led by Samueli School professors Arash Kheradvar and Hamid Jafarkhani, was recently published in the Journal of the Royal Society Interface.
Kheradvar, professor of biomedical engineering and a medical doctor, explains that if 10 cardiologists independently examine an echocardiogram, they will calculate different numbers for the heart chambers’ volume. This may not be very important if the subject’s information is within a normal range, however, if the volume ranges are borderline, this variability can significantly change the course of treatment for a patient. Just a couple percentage points in ejection fraction of a heart chamber could make a difference in the way a patient would be treated, if the number is marginal.
“There have been previous attempts with learning-based medical image analysis, but a major issue in applying artificial intelligence platforms to automatic segmentation of echocardiograms for clinical use is the lack of generalizability,” said Kheradvar.
For example, one can design a very accurate AI algorithm for the dataset that they have, from a hospital or a specific group of patients, but if the model is then used on another dataset, it may not perform well. “A generalizable model is one that can perform well on different datasets, not just the one used in training of the model,” Kheradvar said.
Unlike the standard automated approaches, the UCI researchers’ method is based on learning several levels of representations, corresponding to a hierarchy of features, and not speculating on any model or assumption about the image or heart chambers. The researchers successfully demonstrated the feasibility and performance of their method through computing validation metrics with respect to the gold standard, that of an expert cardiologist.
“To verify our method's generalizability in comparison with other existing techniques, we compared its performance with the state-of-the-art method on our dataset in addition to an independent dataset of 450 patients,” said Jafarkhani, Chancellor’s Professor of electrical engineering and computer science.
The work was the focus of the doctoral dissertation of Arghavan Arafati, the article’s first author, who received her doctorate in mechanical and aerospace engineering from UCI and is currently a research fellow at Harvard Medical School. Other contributors are Dr. Daisuke Morisawa, Hyogo College of Medicine, Japan; Michael R. Avendi, principal scientist at Appriss; Dr. M. Reza Amini, Loma Linda University; and Dr. Ramin A. Assadi, UCLA.
– Lori Brandt