CEE Seminar: Machine Learning for Multiscale Modeling of Heterogeneous Materials
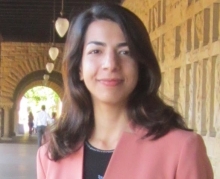
Abstract: Many natural and synthetic materials, e.g. geological and cementitious materials, are multiscale in nature. They typically demonstrate a nonlinear and path-dependent behavior, which is determined by the properties, shape and distribution of the constituents. One of the major challenges associated with multiscale modeling of systems made of these materials includes computational costs of high-fidelity simulations and transferring the information across different length scales. In this talk, we present the recent advances in machine learning-enhanced modeling for path-dependent and multi-physics behavior of heterogeneous materials, which have emerged as a promising alternative to the traditional constitutive models that can enhance simulations of complex systems.
Bio: Shabnam Semnani is an assistant professor in the Department of Structural Engineering at UCSD. Prior to joining UCSD, she earned her doctorate in civil and environmental engineering from Stanford. Semnani is broadly interested in combining experiments, mechanics, computational methods and statistical and machine learning techniques to address critical problems in the infrastructure and sustainability of the built environment. Some of the applications of her work include landslide hazard assessment, earthquake physics, carbon sequestration, geothermal energy production, multiscale material data fusion and advanced infrastructure materials and systems. She has received multiple honors and awards such as ARCS Scholarship and NSF Graduate Research Fellowship.