CEE Seminar: The Science of Complex Systems
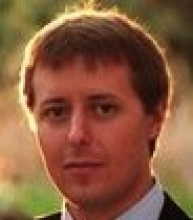
Research Scientist
Hydrological Sciences Lab
NASA Goddard
Faculty
Electrical Engineering & Computer Science
University of Maryland
Abstract:
Model evaluation efforts in the environmental sciences are typically organized around a concept of uncertainty – we try to understand how much error or uncertainty should be associated with model predictions.This approach is incoherent in the sense that any scientific method based on a concept of uncertainty admits at least two distinct logical contradictions (Nearing et al. 2016). Nearing proposes that we should instead be asking questions about information, and that changing our perspective in this particular manner alleviates, or at least attenuates, several longstanding problems that environmental modelers have typically treated as intractable.
In particular, he proposes that an information-centric perspective to model evaluation leads to: (i) purely logical hypothesis tests for complex system models, which addresses the central problem discussed by Neyman (1957), (ii) direct strategies for process-level diagnostics, which addresses the Duhem-Quine problem, and (iii) asymptotically correct estimates of epistemic uncertainty, which addresses the central problem discussed by Knight (1921).
The intuition is that we know from Knuth’s (2005) version of Shannon’s (1948) second theorem that there is a unique quantitative doxastic model of performing a science experiment, and the problem is that this model is missing from all major existing philosophies of science. Adding this model of an experiment to either standard falsificationism or a modern version of inductivism is sufficient to facilitate the resolutions mentioned above. Nearing does not address Hume’s problem, but does reduce its effects to a divergence that reliably approaches epsilon over very large classes of models.
Additionally, this philosophy suggests that we should be building models around maximum entropy distributions instead of as solutions to conservation equations. It turns out that if we do this, we get for free the ability for our models to learn directly from data that was posed as a “Grand Challenge” by Beck (2009). And anyway, given recent results like Best (2015), now may be a good time to rethink our general strategy for building models.
Speaker Bio:
Nearing received his Ph.D. from the University of Arizona under Hoshin Gupta in 2013, and has worked as a contractor in the Hydrological Sciences Lab at NASA Goddard. He currently holds a 50% appointment with NCAR, as well as a research faculty position in the Department of Electrical Engineering and Computer Science at the University of Maryland Baltimore County, where he works on the application of quantum computing to earth science modeling and data assimilation.
References:
Beck, M. B., H. Gupta, E. Rastetter, C. Shoemaker, D. Tarboton, R. Butler, D. Edelson, H. Graber, L. Gross, and T. Harmon. 2009. "Grand challenges of the future for environmental modeling." White Paper, National Science Foundation, Arlington, Virginia.
Best, M. J., G. Abramowitz, H. R. Johnson, A. J. Pitman, G. Balsamo, A. Boone, M. Cuntz, B. Decharme, P. A. Dirmeyer, and J. Dong. 2015. "The plumbing of land surface models: benchmarking model performance." Journal of Hydrometeorology (2015).
Knight, Frank H. 1921. Risk, Uncertainty and Profit. Boston, MA: Hart, Schaffner & Marx; Houghton Mifflin Company.
Knuth, Kevin H. 2005. "Lattice duality: The origin of probability and entropy." Neurocomputing 67:245-274.
Nearing, G. S., Y. Tian, H. V. Gupta, M. P. Clark, K. W. Harrison, and S. V. Weijs. 2016. "A philosophical basis for hydrologic uncertainty." Hydrological Sciences Journal.
Neyman, Jerzy. 1957. "" Inductive Behavior" as a Basic Concept of Philosophy of Science." Revue de l'Institut International de Statistique:7-22.
Shannon, C. E. 1948. "A Mathematical Theory of Communication." Bell System Technical Journal 27 (3):379-423. doi: http://dx.doi.org/10.1063/1.3067010.